Intalytics Q&A – What Operators Can Learn from Outliers
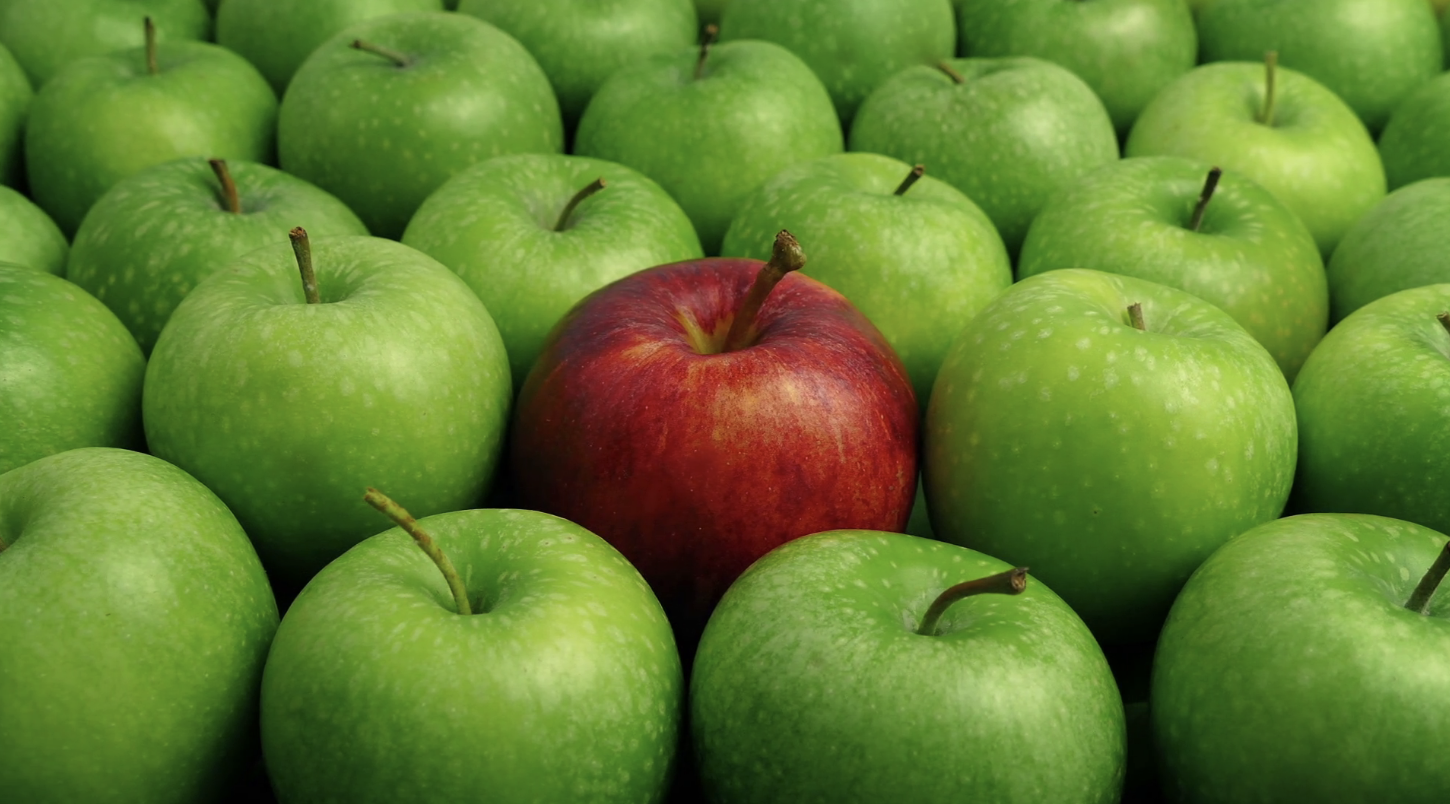
For brands seeking to improve the precision of their site selection strategies, a foundational analytical undertaking is the development of a predictive model to help quantify the potential of any prospective location. In this article, we ask industry experts Justin Tischler and Bill McKeogh to dive deeper into that analytical process – and what operators can learn from studying locations that stand out from the pack.
First things first – what exactly is an “outlier”? Is it just a high- or low-performing location?
Justin Tischler: While a high-performing location can be an outlier, it takes more than just an evaluation of sales history to say that definitively. Oftentimes the sales of high performers can be really well explained by the predictive model. But really what we are talking about here is locations that seem to defy expectations or defy explanation at a glance. Or put another way, these are units that drastically over-perform or under-perform model forecasts.
Bill McKeogh: Any location that significantly differs from the majority, from either a performance or operational standpoint, could be considered an outlier. In most cases, obvious operational outliers would be excluded from the predictive model development process. When defining outliers in the context of a forecasting model, the emphasis often shifts to a focus on the delta between actual performance and model-identified potential.
It sounds like these are locations a model “missed”. You both seem to imply there is a lot to learn from these locations – what can you learn and how do you put it into practice?
JT: It is important to understand that no model is perfect, and there are always going to be locations whose performance defies the patterns of behavior identified elsewhere in the network. That said, locations that a particular model misses can be studied in groups to potentially identify factors that are not in that version of the model, but which might make sense to incorporate in the next iteration. This process is in fact core to how we approach our model development – if the first version of the model under-predicts on average those locations with excellent visibility, then some type of visibility score would be a good candidate variable to introduce into the mix for round two.
BM: There will always be locations whose performance deviates from the modeled potential. As you apply the model against your existing network and identify outliers you might ask, “Why did the model miss these locations?”, but I’d argue the more actionable question is, “Why are my locations exceeding or falling short of their potential?”. A simple flip in the phrasing of the question opens an analytical framework for you to maximize the value of your forecasting model. The one prerequisite is that you trust your model with an adherence to sound statistical practices and guidelines, which I will humbly allow Justin to expand upon…
JT: It is crucial to have the experience to know when to stop adding variables. While most outlier locations are ‘explainable’, it is counterproductive to try to account for every circumstance in the model – either you will have a problem measuring all the relevant inputs, or the sample sizes will get unmanageably small – in either case, compromising the model’s ability to work well on prospective trade areas. I recall working with a client in the healthcare space evaluating their locations that under-performed against our model, and each one had a rational, but difficult-to-measure, reason for poorer than expected performance. For example, “the only doctor at this location got stuck out of the country for 4 months” is not worth trying to fit into the model.
BM: The primary objective of a predictive model is to be predictive. If the focus shifts towards an identification of every nuanced attribute that has historically impacted performance, you will have lost the forest for the trees. Outliers are a valuable tool in the overall site selection process, and a thorough review of both over- and under-performing locations offers an immense opportunity for learning.
What other recommendations would you provide to someone studying outliers in the results of their predictive model?
JT: Focus on the trends. It is easy to get caught up talking about one or two locations – but what is really important is identifying whether locations that share a common attribute systematically over- or under-perform expectations – that is an insight that is actionable and will make the next model perform better, with the added benefit of helping you understand its biases in the meantime.
BM: I agree with Justin here, it is really important to identify the common denominators associated with outliers and recognize if that attribute is accounted for in the modeling process. Start to consider what data you may need to collect or procure to help validate an emerging hypothesis. Remember that you do not have to define outliers through a single lens. In some cases, the actual deviation may be less important than the directional deviation.
Finally, I just want to emphasize how useful an outlier review can be across the organization, from identifying relocation candidates or allocation of capital reinvestment expenditure to supporting marketing spend or in-store merchandising decisions – the list goes on and on.
To learn more about how Intalytics partners with brands to identify what drives success in their real estate portfolios and marketing campaigns, please contact us.
Related News
Carousel items